
Most of these studies used listwise deletion, because it once was a standard way to deal with missings and still is in many software packages. As an example, Ranjit Lall examined how political science studies dealed with missing data and found out, that 50 % had their key results „disappear“ after he re-analysed them with a proper way to handle the missingness: How multiple Imputation makes a difference. But there would be a lot reason to pay more attention to this issue. Also in practical research a lot of studies don´t show transparently how they handled missing data. The topic of missing data itself is still often missing in the curriculum of statistics for social sciences and sociology. Item nonresponse occurs, when a person leaves out particular items in the survey, or – more generally spoken – particular measurements of a sampled unit are missing. There are two types of missingness: Unit nonresponse concerns cases in the sample, that didn´t respond to the survey at all, or – more generally spoken – the failure to obtain measurements for a sampled unit. If you are in a hurry and already know the background of multiple imputation, jump to: How to use multiple imputation with lavaan The following post will give an overview on the background of missing data analysis, how the missingness can be investigated, how the R-package MICE for multiple imputation is applied and how imputed data can be given to the lavaan-package for confirmatory factor analysis.
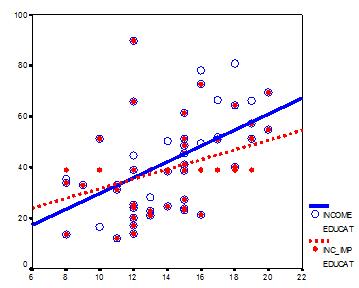
Structural equation modeling and confirmatory factor analysis are such methods that rely on a complete dataset. This can be a problem for any statistical analysis that needs data to be complete. Missing data is unavoidable in most empirical work.
